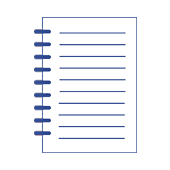
Application of Machine Learning Methods for an Analysis of E-Nose Multidimensional Signals in Wastewater Treatment
Artykuł w czasopiśmie
MNiSW
100
Lista 2023
Status: | |
Autorzy: | Piłat-Rożek Magdalena, Łazuka Ewa, Majerek Dariusz, Szeląg Bartosz, Duda-Saternus Sylwia, Łagód Grzegorz |
Dyscypliny: | |
Aby zobaczyć szczegóły należy się zalogować. | |
Rok wydania: | 2023 |
Wersja dokumentu: | Drukowana | Elektroniczna |
Język: | angielski |
Numer czasopisma: | 1 |
Wolumen/Tom: | 23 |
Numer artykułu: | 487 |
Strony: | 1 - 18 |
Impact Factor: | 3,4 |
Web of Science® Times Cited: | 14 |
Scopus® Cytowania: | 23 |
Bazy: | Web of Science | Scopus | ADS | CABI CAB Direct CAPlus / SciFinder CNKI dblp Computer Science Bibliography DOAJ | EBSCO | INSPIRE MEDLINE PMC OpenAIRE OSTI | PATENTSCOPE | ProQuest |
Efekt badań statutowych | NIE |
Materiał konferencyjny: | NIE |
Publikacja OA: | TAK |
Licencja: | |
Sposób udostępnienia: | Witryna wydawcy |
Wersja tekstu: | Ostateczna wersja opublikowana |
Czas opublikowania: | W momencie opublikowania |
Data opublikowania w OA: | 2 stycznia 2023 |
Abstrakty: | angielski |
The work represents a successful attempt to combine a gas sensors array with instrumentation (hardware), and machine learning methods as the basis for creating numerical codes (software), together constituting an electronic nose, to correct the classification of the various stages of the wastewater treatment process. To evaluate the multidimensional measurement derived from the gas sensors array, dimensionality reduction was performed using the t-SNE method, which (unlike the commonly used PCA method) preserves the local structure of the data by minimizing the Kullback-Leibler divergence between the two distributions with respect to the location of points on the map. The k-median method was used to evaluate the discretization potential of the collected multidimensional data. It showed that observations from different stages of the wastewater treatment process have varying chemical fingerprints. In the final stage of data analysis, a supervised machine learning method, in the form of a random forest, was used to classify observations based on the measurements from the sensors array. The quality of the resulting model was assessed based on several measures commonly used in classification tasks. All the measures used confirmed that the classification model perfectly assigned classes to the observations from the test set, which also confirmed the absence of model overfitting. |