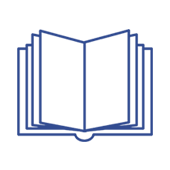
Fuzzy c-means based extended isolation forest for anomaly detection
Fragment książki (Rozdział monografii pokonferencyjnej)
MNiSW
20
Poziom I
Status: | |
Autorzy: | Ayoub Mniai, Khalid Jebari, Karczmarek Paweł |
Dyscypliny: | |
Aby zobaczyć szczegóły należy się zalogować. | |
Wersja dokumentu: | Drukowana | Elektroniczna |
Język: | angielski |
Strony: | 411 - 418 |
Scopus® Cytowania: | 2 |
Bazy: | Scopus |
Efekt badań statutowych | NIE |
Materiał konferencyjny: | TAK |
Nazwa konferencji: | International Conference on Advanced Intelligent Systems for Sustainable Development 2022 |
Skrócona nazwa konferencji: | AI2SD 2022 |
URL serii konferencji: | LINK |
Termin konferencji: | 22 maja 2022 do 27 maja 2022 |
Miasto konferencji: | Rabat |
Państwo konferencji: | MAROKO |
Publikacja OA: | NIE |
Abstrakty: | angielski |
Anomaly detection is a mechanism that identifies different observations from regular instances. Also, it is often applied to unlabeled data, which is known as unsupervised anomaly detection. In the last decade, many research studies have provided techniques that enhance and improve the quality of anomaly detection. Unfortunately, they are not very accurate for high-dimensional data. Also, the exponential growth in data volume and complexity generated by modern applications affects existing techniques in terms of both performance and accuracy. However, a modified version of the Isolation Forest (IF), Extended Isolation Forest (EIF), has improved the robustness of the anomaly score map. The EIF can build scores with less variance and obtain better accuracy. This study presents an enhanced version of the Extended Isolation Forest algorithm. This is a Fuzzy C-Means-Based Extended Isolation Forest (FCMBEIF), which allows building the search trees and nodes based on Fuzzy C-Means (FCM) clustering. Experimental results demonstrate the performance of the proposed algorithm and show that FCM clustering contributes to the algorithm’s efficiency. |