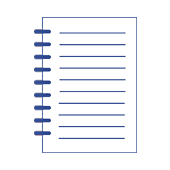
Application of Fuzzy Neural Networks in Combustion Process Diagnostics
Artykuł w czasopiśmie
MNiSW
140
Lista 2024
Status: | |
Autorzy: | Grądz Żaklin, Wójcik Waldemar, Gromaszek Konrad, Kotyra Andrzej, Smailova Saule, Iskakova Aigul, Yeraliyeva Bakhyt, Kumargazhanova Saule, Imanbek Baglan |
Dyscypliny: | |
Aby zobaczyć szczegóły należy się zalogować. | |
Rok wydania: | 2024 |
Wersja dokumentu: | Drukowana | Elektroniczna |
Język: | angielski |
Numer czasopisma: | 1 |
Wolumen/Tom: | 17 |
Numer artykułu: | 212 |
Strony: | 1 - 19 |
Impact Factor: | 3,0 |
Web of Science® Times Cited: | 0 |
Scopus® Cytowania: | 0 |
Bazy: | Web of Science | Scopus |
Efekt badań statutowych | NIE |
Materiał konferencyjny: | NIE |
Publikacja OA: | TAK |
Licencja: | |
Sposób udostępnienia: | Witryna wydawcy |
Wersja tekstu: | Ostateczna wersja opublikowana |
Czas opublikowania: | W momencie opublikowania |
Data opublikowania w OA: | 30 grudnia 2023 |
Abstrakty: | angielski |
Coal remains one of the key raw materials used in the energy industry to generate electricity and heat. As a result, diagnostics of the combustion process is still an important topic of scientific research. Correct implementation of the process allows the emission of pollutants into the atmosphere to be kept at a compliant level. Therefore, it is important to conduct the process in a manner that will not exceed these standards. A preliminary analysis of the measurement signals was carried out, and signal predictions of flame intensity changes were determined using the autoregressive moving average (ARMA) model. Different fuzzy neural network architectures have been investigated. Binary and multi-class classifications of flame states were conducted. The best results were obtained from the ANFIS_grid partition model, producing an accuracy of 95.46% for binary classification and 79.08% for multi-class classification. The accuracy of the recognition of flame states and the high convergence of the determined predictions with measurement signals validate the application of the proposed approach in diagnosing or controlling the combustion process of pulverized coal and its mixtures with biomass. Expert decisions determine the range of acceptable states. |