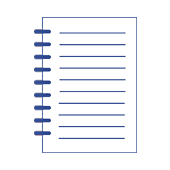
Microscopic Studies of Activated Sludge Supported by Automatic Image Analysis Based on Deep Learning Neural Networks
Artykuł w czasopiśmie
MNiSW
70
Lista 2024
Status: | |
Autorzy: | Dziadosz Marcin, Majerek Dariusz, Łagód Grzegorz |
Dyscypliny: | |
Aby zobaczyć szczegóły należy się zalogować. | |
Rok wydania: | 2024 |
Wersja dokumentu: | Drukowana | Elektroniczna |
Język: | angielski |
Numer czasopisma: | 4 |
Wolumen/Tom: | 25 |
Strony: | 360 - 369 |
Impact Factor: | 1,3 |
Scopus® Cytowania: | 0 |
Bazy: | Scopus | BazTech |
Efekt badań statutowych | NIE |
Finansowanie: | Fundusz dyscypliny Inżynieria Środowiska, Górnictwo i Energetyka |
Materiał konferencyjny: | NIE |
Publikacja OA: | TAK |
Licencja: | |
Sposób udostępnienia: | Otwarte czasopismo |
Wersja tekstu: | Ostateczna wersja opublikowana |
Czas opublikowania: | Po opublikowaniu |
Data opublikowania w OA: | 1 marca 2024 |
Abstrakty: | angielski |
Paper presents a microscopic studies of activated sludge supported by automatic image analysis based on deep learning neural networks. The organisms classified as Arcella vulgaris were chosen for the research. They frequently occur in the waters containing organic substances as well as WWTPs employing the activated sludge method. Usually, they can be clearly seen and counted using a standard optical microscope, as a result of their distinctive appearance, numerous population and passive behavior. Thus, these organisms constitute a viable object for detection task. Paper refers to the comparison of performance of deep learning networks namely YOLOv4 and YOLOv8, which conduct automatic image analysis of the afore-mentioned organisms. YOLO constitutes a one-stage object detection model that look at the analyzed image once and allow real-time detection without a marked accuracy loss. The training of the applied YOLO models was carried out using sample microscopic images of activated sludge. The relevant training data set was created by manually labeling the digital images of organisms, followed by calculation and comparison of various metrics, including recall, precision, and accuracy. The architecture of the networks built for the detection task was general, which means that the structure of the layers and filters was not affected by the purpose of using the models. Accounting mentioned universal construction of the models, the results of the accuracy and quality of the classification can be considered as very good. This means that the general architecture of the YOLO networks can also be used for specific tasks such as identification of shell amoebas in activated sludge. |