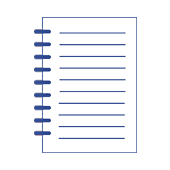
Gearbox fault identification using auto-encoder without training data from the damaged machine
Artykuł w czasopiśmie
MNiSW
200
Lista 2024
Status: | |
Autorzy: | Pawlik Paweł, Kania Konrad, Przysucha Bartosz |
Dyscypliny: | |
Aby zobaczyć szczegóły należy się zalogować. | |
Rok wydania: | 2024 |
Wersja dokumentu: | Drukowana | Elektroniczna |
Język: | angielski |
Numer czasopisma: | Pt A |
Wolumen/Tom: | 242 |
Numer artykułu: | 115805 |
Strony: | 1 - 20 |
Impact Factor: | 5,2 |
Web of Science® Times Cited: | 0 |
Scopus® Cytowania: | 0 |
Bazy: | Web of Science | Scopus |
Efekt badań statutowych | NIE |
Finansowanie: | This work was supported by the Polish Ministry of Science and Higher Education [Grants numbers 16.16.130.942, FD-20/IM-5/088] |
Materiał konferencyjny: | NIE |
Publikacja OA: | TAK |
Licencja: | |
Sposób udostępnienia: | Witryna wydawcy |
Wersja tekstu: | Ostateczna wersja opublikowana |
Czas opublikowania: | Przed opublikowaniem |
Data opublikowania w OA: | 26 września 2024 |
Abstrakty: | angielski |
Deep learning methods work well in machine diagnostics where operating conditions affect diagnostic signals. Classifiers are often used for fault identification, but these methods require training data sets measured for each fault. The solution to the lack of data is autoencoder-based network models, but these models can only detect, not identify faults. This article presents a new fault identification method based on auto-encoders (AE-based Fault Identification Technique AE-FIT) that does not require training data from the damaged machine. This method diagnoses pinion gearboxes operating under variable conditions (variable load, load-induced rotational speed, and temperature). The result of the technique is an interpretable diagnostic spectrum (AE-based Interpretable Order Spectrum AEIOS). The method has been tested on two laboratory benches to detect misalignment, unbalance, and gearbox degradation. The damages introduced were used to validate a technique based on an auto-encoder trained only with data from undamaged machines. |