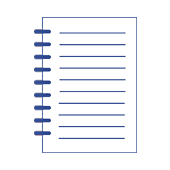
Automatic System for Acquisition and Analysis of Microscopic Digital Images Containing Activated Sludge
Artykuł w czasopiśmie
MNiSW
100
Lista 2024
Status: | |
Autorzy: | Staniszewski Michał, Dziadosz Marcin, Zaburko Jacek, Babko Roman, Łagód Grzegorz |
Dyscypliny: | |
Aby zobaczyć szczegóły należy się zalogować. | |
Rok wydania: | 2024 |
Wersja dokumentu: | Drukowana | Elektroniczna |
Język: | angielski |
Numer czasopisma: | 7 |
Wolumen/Tom: | 18 |
Strony: | 51 - 61 |
Impact Factor: | 1,0 |
Bazy: | BazTech |
Efekt badań statutowych | NIE |
Finansowanie: | Z Funduszu Dyscypliny FD 20/IS-6/50 |
Materiał konferencyjny: | NIE |
Publikacja OA: | TAK |
Licencja: | |
Sposób udostępnienia: | Otwarte czasopismo |
Wersja tekstu: | Ostateczna wersja opublikowana |
Czas opublikowania: | W momencie opublikowania |
Data opublikowania w OA: | 6 października 2024 |
Abstrakty: | angielski |
The article contains the procedure of image acquisition, including sampling of analyzed material as well as technical solutions of hardware and preprocessing used in research. A dataset of digital images containing identified objects were obtained with help of automated mechanical system for controlling the microscope table and used to train the YOLO models. The performance of YOLOv4 as well as YOLOv8 deep learning networks was compared on the basis of automatic image analysis. YOLO constitutes a one-stage object detection model, aiming to examine the analyzed image only once. By utilizing a single neural network, the image is divided into a grid of cells, and predictions are made for bounding boxes, as well as object class probabilities for each box. This approach allows real-time detection with minimal accuracy loss. The study involved ciliated protozoa Vorticella as a test object. These organisms are found both in natural water bodies and in treatment plants that employ the activated sludge method. As a result of its distinct appearance, high abundance and sedentary lifestyle, Vorticella are good subjects for detection tasks. To ensure that the training dataset is accurate, the images were manually labeled. The performance of the models was evaluated using such metrics as accuracy, precision, and recall. The final results show the differences in metrics characterizing the obtained outputs and progress in the software over subsequent versions of the YOLO algorithm. |