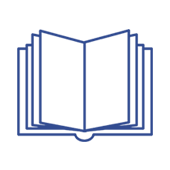
The Concept of Detecting and Classifying Anomalies in Large Data Sets on a Basis of Information Granules
Fragment książki (Materiały konferencyjne)
MNiSW
140
konferencja
Status: | |
Autorzy: | Kiersztyn Adam, Karczmarek Paweł, Kiersztyn Krystyna, Pedrycz Witold |
Dyscypliny: | |
Aby zobaczyć szczegóły należy się zalogować. | |
Wersja dokumentu: | Drukowana | Elektroniczna |
Język: | angielski |
Strony: | 1 - 7 |
Web of Science® Times Cited: | 15 |
Scopus® Cytowania: | 16 |
Bazy: | Web of Science | Scopus | IEEE Xplore |
Efekt badań statutowych | NIE |
Finansowanie: | Funded by the National Science Centre, Poland under CHIST-ERA programme (Grant no. 2018/28/Z/ST6/00563). |
Materiał konferencyjny: | TAK |
Nazwa konferencji: | IEEE International Conference onFuzzy Systems (FUZZ-IEEE) 2020 |
Skrócona nazwa konferencji: | FUZZ-IEEE 2020 |
URL serii konferencji: | LINK |
Termin konferencji: | 19 lipca 2020 do 24 lipca 2020 |
Miasto konferencji: | Glasgow |
Państwo konferencji: | WIELKA BRYTANIA |
Publikacja OA: | NIE |
Abstrakty: | angielski |
Anomaly (outlier) detection is one of the most important problems of modern data analysis. Anomalies can be the results of database users' mistakes, operational errors or just missing values. The problem is important because of fast growth of the large data sets. Therefore, we present the initial results of work on a Granular Computing approach to data imputation and missing data analysis. Our proposal brings intuitive and interpretable solutions. Finally, in a series of experiments, we demonstrate its effectiveness for a large dataset in the area of transport. |