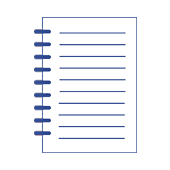
Detection and Classification of Anomalies in Large Data Sets on the Basis of Information Granules
Artykuł w czasopiśmie
MNiSW
200
Lista 2021
Status: | |
Autorzy: | Kiersztyn Adam, Karczmarek Paweł, Kiersztyn Krystyna, Pedrycz Witold |
Dyscypliny: | |
Aby zobaczyć szczegóły należy się zalogować. | |
Rok wydania: | 2022 |
Wersja dokumentu: | Drukowana | Elektroniczna |
Język: | angielski |
Numer czasopisma: | 8 |
Wolumen/Tom: | 30 |
Strony: | 2850 - 2860 |
Impact Factor: | 11,9 |
Web of Science® Times Cited: | 11 |
Scopus® Cytowania: | 13 |
Bazy: | Web of Science | Scopus |
Efekt badań statutowych | NIE |
Finansowanie: | This work was supported by the National Science Centre, Poland under CHIST-ERA programme under Grant 2018/28/Z/ST6/00563. |
Materiał konferencyjny: | NIE |
Publikacja OA: | NIE |
Abstrakty: | angielski |
Anomaly (outlier) detection is one of the most important problems of modern data analysis. The sources of anomalies are varying. They can be the results of database users' mistakes, operational errors or just missing values. The problem is very important because of the fast growth of large data sets. Therefore, in this study, we present detailed results of work on the concept of Granular Computing-based approach to anomaly detection, classification, and gradation. The aim of the study is to introduce an innovative solution that allows the use of information granules to identify and classify anomalies. The novelty of the proposed solution consists in the use of fuzzy semantics implied by the statistical properties of the data considered. Moreover, instead of the classic approach to detecting anomalies in the data, it is proposed to determine the degree of anomaly for the data transformed to the new resulting state space. Thanks to the use of an innovative approach using the universal descriptor space, it is possible to determine the degree of anomaly, and by using various aggregation methods one can also specify its type. |