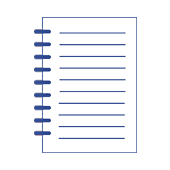
Logistic Regression with Wave Preprocessing to Solve Inverse Problem in Industrial Tomography for Technological Process Control
Artykuł w czasopiśmie
MNiSW
140
Lista 2021
Status: | |
Autorzy: | Rymarczyk Tomasz, Niderla Konrad, Kozłowski Edward, Król Krzysztof, Wyrwisz Joanna, Skrzypek-Ahmed Sylwia, Gołąbek Piotr |
Dyscypliny: | |
Aby zobaczyć szczegóły należy się zalogować. | |
Rok wydania: | 2021 |
Wersja dokumentu: | Drukowana | Elektroniczna |
Język: | angielski |
Numer czasopisma: | 23 |
Wolumen/Tom: | 14 |
Numer artykułu: | 8116 |
Strony: | 1 - 20 |
Impact Factor: | 3,252 |
Web of Science® Times Cited: | 10 |
Scopus® Cytowania: | 11 |
Bazy: | Web of Science | Scopus |
Efekt badań statutowych | NIE |
Materiał konferencyjny: | NIE |
Publikacja OA: | TAK |
Licencja: | |
Sposób udostępnienia: | Witryna wydawcy |
Wersja tekstu: | Ostateczna wersja opublikowana |
Czas opublikowania: | W momencie opublikowania |
Data opublikowania w OA: | 3 grudnia 2021 |
Abstrakty: | angielski |
The research presented here concerns the analysis and selection of logistic regression with wave preprocessing to solve the inverse problem in industrial tomography. The presented application includes a specialized device for tomographic measurements and dedicated algorithms for image reconstruction. The subject of the research was a model of a tank filled with tap water and specific inclusions. The research mainly targeted the study of developing and comparing models and methods for data reconstruction and analysis. The application allows choosing the appropriate method of image reconstruction, knowing the specifics of the solution. The novelty of the presented solution is the use of original machine learning algorithms to implement electrical impedance tomography. One of the features of the presented solution was the use of many individually trained subsystems, each of which produces a unique pixel of the final image. The methods were trained on data sets generated by computer simulation and based on actual laboratory measurements. Conductivity values for individual pixels are the result of the reconstruction of vector images within the tested object. By comparing the results of image reconstruction, the most efficient methods were identified. |