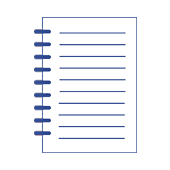
Ultrasound tomography enhancement by signal feature extraction with modular machine learning method
Artykuł w czasopiśmie
MNiSW
100
Lista 2024
Status: | |
Autorzy: | Baran Bartłomiej, Majerek Dariusz, Szyszka Piotr, Wójcik Dariusz, Rymarczyk Tomasz |
Dyscypliny: | |
Aby zobaczyć szczegóły należy się zalogować. | |
Rok wydania: | 2024 |
Wersja dokumentu: | Drukowana | Elektroniczna |
Język: | angielski |
Numer czasopisma: | 1 |
Wolumen/Tom: | 19 |
Numer artykułu: | e0297496 |
Strony: | 1 - 13 |
Impact Factor: | 2,9 |
Web of Science® Times Cited: | 0 |
Scopus® Cytowania: | 0 |
Bazy: | Web of Science | Scopus | Google Scholar |
Efekt badań statutowych | NIE |
Materiał konferencyjny: | NIE |
Publikacja OA: | TAK |
Licencja: | |
Sposób udostępnienia: | Otwarte czasopismo |
Wersja tekstu: | Ostateczna wersja opublikowana |
Czas opublikowania: | W momencie opublikowania |
Data opublikowania w OA: | 31 stycznia 2024 |
Abstrakty: | angielski |
Robust and reliable diagnostic methods are desired in various types of industries. This article presents a novel approach to object detection in industrial or general ultrasound tomography. The key idea is to analyze the time-dependent ultrasonic signal recorded by three independent transducers of an experimental system. It focuses on finding common or related characteristics of these signals using custom-designed deep neural network models. In principle, models use convolution layers to extract common features of signals, which are passed to dense layers responsible for predicting the number of objects or their locations and sizes. Predicting the number and properties of objects are characterized by a high value of the coefficient of determination R2 = 99.8% and R2 = 98.4%, respectively. The proposed solution can result in a reliable and low-cost method of object detection for various industry sectors. |