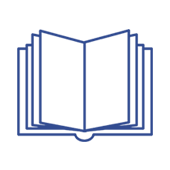
Improving Image Reconstruction Quality in Ultrasonic Tomography Using Deep Neural Networks
Fragment książki (Materiały konferencyjne)
MNiSW
200
konferencja
Status: | |
Autorzy: | Kulisz Monika, Kłosowski Grzegorz, Rymarczyk Tomasz, Niderla Konrad, Bednarczuk Piotr |
Dyscypliny: | |
Aby zobaczyć szczegóły należy się zalogować. | |
Wersja dokumentu: | Drukowana | Elektroniczna |
Język: | angielski |
Strony: | 520 - 521 |
Web of Science® Times Cited: | 0 |
Scopus® Cytowania: | 0 |
Bazy: | Web of Science | Scopus | dblp |
Efekt badań statutowych | NIE |
Materiał konferencyjny: | TAK |
Nazwa konferencji: | 21st ACM Conference on Embedded Networked Sensor Systems |
Skrócona nazwa konferencji: | 21st SenSys 2023 |
URL serii konferencji: | LINK |
Termin konferencji: | 12 listopada 2023 do 17 listopada 2023 |
Miasto konferencji: | Istanbul |
Państwo konferencji: | TURCJA |
Publikacja OA: | TAK |
Licencja: | |
Sposób udostępnienia: | Witryna wydawcy |
Wersja tekstu: | Ostateczna wersja opublikowana |
Czas opublikowania: | W momencie opublikowania |
Data opublikowania w OA: | 26 kwietnia 2024 |
Abstrakty: | angielski |
This study aims to improve the resolution of reconstructed images from industrial ultrasonic tomography (UST) by determining the most effective neural network structure for solving the inverse problem based on the measurements taken. The study analyzed three types of neural networks: Artificial Neural Networks, Convolutional Neural Networks (CNNs) and a hybrid of CNNs and Long Short-Term Memory networks (LSTM). After evaluating the reconstructions and quality indicators, the CNN-LSTM combination provided the most accurate image reconstructions of the industrial ultrasound tomography, highlighting the importance of selecting an appropriate neural network to improve the resolution of the reconstructed images. |