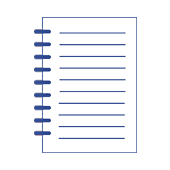
The Effect of the Context Length of Large Language Models on the Quality of Extracted Information in Polish in the Process of Project Management
Artykuł w czasopiśmie
MNiSW
100
Lista 2024
Status: | |
Autorzy: | Pliszczuk Damian, Maj Michał, Marek Patryk, Wilczewska Weronika, Cieplak Tomasz, Rymarczyk Tomasz |
Dyscypliny: | |
Aby zobaczyć szczegóły należy się zalogować. | |
Rok wydania: | 2024 |
Wersja dokumentu: | Drukowana | Elektroniczna |
Język: | angielski |
Numer czasopisma: | 3 |
Wolumen/Tom: | 27 |
Strony: | 414 - 425 |
Efekt badań statutowych | NIE |
Materiał konferencyjny: | NIE |
Publikacja OA: | TAK |
Licencja: | |
Sposób udostępnienia: | Otwarte czasopismo |
Wersja tekstu: | Ostateczna wersja opublikowana |
Czas opublikowania: | W momencie opublikowania |
Data opublikowania w OA: | 30 października 2024 |
Abstrakty: | angielski |
Purpose: This paper presents GraphRAG, a novel tool that integrates large language models (LLMs) with knowledge graphs to enhance the precision and consistency of responses generated from unstructured text data. The primary objective is to improve the quality of information retrieval and synthesis for complex user queries requiring comprehensive understanding. Desugn/Methodology/Approach: The GraphRAG framework processes source documents by dividing them into smaller fragments (chunks) to facilitate knowledge extraction. Using community detection algorithms, such as the Leiden algorithm, GraphRAG identifies semantic clusters within the knowledge graph, enabling both local and global information retrieval. The tool employs a multi-stage analysis approach, leveraging prompts to detect entities and relationships in the text, which are then organized into structured graph nodes and edges. Findings: The experimental results reveal that smaller chunk sizes (e.g., 300 tokens) significantly improve the granularity of detected entities and relationships, leading to a more detailed knowledge graph structure. This approach enhances response accuracy for knowledge-intensive queries by enabling the LLM to focus on specific text segments, improving the precision of extracted information. Practical Implications: GraphRAG has practical applications in any domain where accurate and context-rich responses are essential, such as customer support, decision-making, and research analysis. By balancing chunk size and processing efficiency, the tool enables scalable analysis while maintaining high data quality, making it a valuable asset for knowledge-intensive tasks. Originality/Value: This research contributes to the field by demonstrating an effective integration of LLMs with knowledge graphs to process large text corpora. GraphRAG’s method of combining local and global retrieval through knowledge graphs represents an advancement over traditional retrieval-augmented generation methods, especially in scenarios requiring detailed information synthesis. |