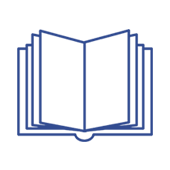
The Concept of an Ultrasensitive Industrial Ultrasound Scanner Using Hilbert and Wavelet Transforms in a Machine Learning Model
Fragment książki (Materiały konferencyjne)
MNiSW
200
konferencja
Status: | |
Autorzy: | Kłosowski Grzegorz, Rymarczyk Tomasz, Soleimani Manuchehr, Niderla Konrad |
Dyscypliny: | |
Aby zobaczyć szczegóły należy się zalogować. | |
Wersja dokumentu: | Drukowana | Elektroniczna |
Język: | angielski |
Strony: | 873 - 874 |
Efekt badań statutowych | NIE |
Materiał konferencyjny: | TAK |
Nazwa konferencji: | 22nd ACM Conference on Embedded Networked Sensor Systems |
Skrócona nazwa konferencji: | 22nd SenSys 2024 |
URL serii konferencji: | LINK |
Termin konferencji: | 4 listopada 2024 do 7 listopada 2024 |
Miasto konferencji: | Hangzhou |
Państwo konferencji: | CHINY |
Publikacja OA: | TAK |
Licencja: | |
Sposób udostępnienia: | Witryna wydawcy |
Wersja tekstu: | Ostateczna wersja opublikowana |
Czas opublikowania: | W momencie opublikowania |
Data opublikowania w OA: | 4 listopada 2024 |
Abstrakty: | angielski |
The main goal of the research was to develop an effective, highresolution tomographic apparatus capable of non-invasively capturing real-time internal images of industrial tank reactors. For this purpose, a prototype of an ultrasonic tomograph (UST) was developed, which combines innovative design solutions and modern algorithmic techniques. A special feature of the presented solution is the use of a neural network with an unusual architecture. A deep, multi-branch neural network consisting of two inputs was used. The first input is a 120-element vector (sequence) of raw measurements. The third input consists of three sequences obtained as a result of the transformation of raw measurements: instantenous frequency (IF), approximation coefficients (Ca), and detail coefficients (Cd). The prototype was tested on a real model. The tomographic reconstructions obtained using the innovative neural architecture were compared with images obtained using a standard neural network. The results clearly confirm the high effectiveness of the presented approach. |