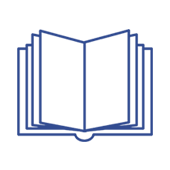
The Use of Machine Learning in Electrical Impedance Tomography - A Variable Frequency Approach
Fragment książki (Materiały konferencyjne)
MNiSW
200
konferencja
Status: | |
Autorzy: | Kulisz Monika, Rymarczyk Tomasz, Kłosowski Grzegorz, Niderla Konrad, Oleszek Michał |
Dyscypliny: | |
Aby zobaczyć szczegóły należy się zalogować. | |
Wersja dokumentu: | Drukowana | Elektroniczna |
Język: | angielski |
Strony: | 830 - 831 |
Efekt badań statutowych | NIE |
Materiał konferencyjny: | TAK |
Nazwa konferencji: | 22nd ACM Conference on Embedded Networked Sensor Systems |
Skrócona nazwa konferencji: | 22nd SenSys 2024 |
URL serii konferencji: | LINK |
Termin konferencji: | 4 listopada 2024 do 7 listopada 2024 |
Miasto konferencji: | Hangzhou |
Państwo konferencji: | CHINY |
Publikacja OA: | TAK |
Licencja: | |
Sposób udostępnienia: | Witryna wydawcy |
Wersja tekstu: | Ostateczna wersja opublikowana |
Czas opublikowania: | W momencie opublikowania |
Data opublikowania w OA: | 4 listopada 2024 |
Abstrakty: | angielski |
This study presents a novel technique for reconstructing the internal structures of industrial tank reactors using electrical impedance tomography (EIT). The method uses three different measurement vectors, each corresponding to different electrical frequencies---100 kHz, 50 kHz, and 10 kHz---to improve the accuracy and reliability of EIT reconstructions. The goal was to get the most out of both the resistive and reactive data from the EIT system by using machine learning methods that took frequency-specific data into account. This data was shown as complex numbers. To process the multi-frequency data collected from the measurements, an LSTM network was used. The results show that the multi-frequency model significantly outperforms single-frequency methods in terms of reconstruction accuracy. |